MEASURING WIND CREATING NEW MODELS TO GAUGE IMPACT ON WILDFIRE
BY BRIAN POTTER, KARA YEDINAK, AND JOSEPH CHARNEY
Few things can raise more concern on a wildland fire than unexpected or uncertain winds. Wind is a primary driver of fire spread in wildland fires, whether wildfires or prescribed burns. Unanticipated fire spread or spotting can result from unexpected changes in wind speed or direction, a change in the timing of a predicted shift, or unexpected variability in wind speed and direction along an active fire front.
Wind played a key role in the escape of the Las Dispensas prescribed burn in New Mexico, which became the Hermits’ Peak fire in early 2022. In response to that escape, the US Forest Service conducted a review of its prescribed burning practices. Wind came up often in discussions by the review teams and featured in several recommendations in the final report. These discussions prompted questions about how wind is measured and modeled, and how scientists and operational meteorologists communicate that information to prescribed fire practitioners. Similar questions have been raised by fire behavior analysts and meteorologists working on wildfires.
In May 2023, in Minneapolis, Minnesota, there was an informal workshop on wind measurement and modeling for wildland fire. Attendees included research meteorologists, operational forecasters from the National Weather Service and National Predictive Services, and academic meteorologists.
The workshop’s goal was to begin exploring:
• how we measure wind for fire
• how we model the impact of wind on fire
• how to interpret numerically modeled winds in the context of fire and observed winds
• whether current practices adequately meet the needs of fire practitioners, for both prescribed fires and wildfires
• whether those practices could be improved, even if they meet current needs.
What follows are examples of concerns raised during the workshop, suggestions for possible research projects, opportunities for better or new tool development, and improved educational content about wind for fire management. The ideas and examples range from the rather philosophical to the highly pragmatic. Because of the nature of the discussions, no idea came from one specific person, and we consider the examples to be community brainchildren. We use “fire management” to refer to both prescribed fire and wildfire situations. If a point applies only to one, we will note that. References to tools and systems are U.S.-centric, but the concepts are intended to apply globally.
Operational analysts and research scientists spend a great deal of effort improving fire behavior models. Yet those improvements are less effective if the wind information they rely on is unrepresentative or poorly defined.
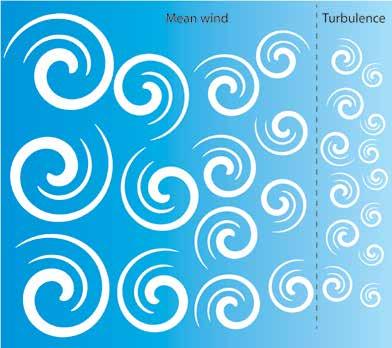
SCALES OF TIME AND SPACE, TURBULENCE VERSUS MEAN
Louis Fry Richardson, the physicist behind the Richardson Number, wrote: “Big whirls have little whirls, that feed on their velocity. And little whirls have lesser whirls, and so on to viscosity.”
All wind is a whirl of air on some scale. We can characterize whirls of different scales by averaging wind measurements over a specific time period. A long period average is more representative of larger, longer duration whirls, and the longer the averaging period, the less it reflects the smaller whirls. However, the variability of the winds around that mean reflects the smaller whirls. Hence, the period of averaging determines what constitutes mean wind, and the variability we call turbulence or gustiness. To the best of the knowledge of those at the workshop, current meteorological practices for averaging periods are based on historic maritime, modern aviation needs, and instrument design limitations.
The choice of averaging period is critical, but it’s not something often considered or discussed. Both the mean wind and the turbulence are important, but in different ways. If the averaging period is very short, the average wind will change quickly, perhaps too quickly for actual use of the information. Fire crews cannot change plans every five minutes based on new wind estimates – even the volume of that information would be detrimental. If the averaging period is very long, it may fail to capture changes (such as gusts or shifts in direction) occurring on time scales that matter for managers. Once-a-day wind values, for example, would be useless and uninformative for most management decisions. For fire applications, the averaging period should reflect the time and space scales of physical fire processes and fire management activities. Exploring the question of the “right” scales for averaging for fire management decisions is one area for research.
MEASURING WIND
The question, “How do we measure the wind?” is guided by those scale considerations. Even within the scope of fire management, different measures are appropriate for aviation, surface fire spread, canopy spread, spotting distance, and smoke dispersion. Considering only surface fire spread, to illustrate further, several factors matter: height of measurement; sampling period; sampling frequency; gust characterization; and perhaps others. Remote Automated Weather Stations (RAWS) report a 10-minute average of six-second samples and a gust speed based on the maximum six-second sample from the previous hour. That convention is based on the limitations of decades-old anemometer designs, and there are numerous new instruments available that could be more helpful for fire management, such as sonic anemometers.
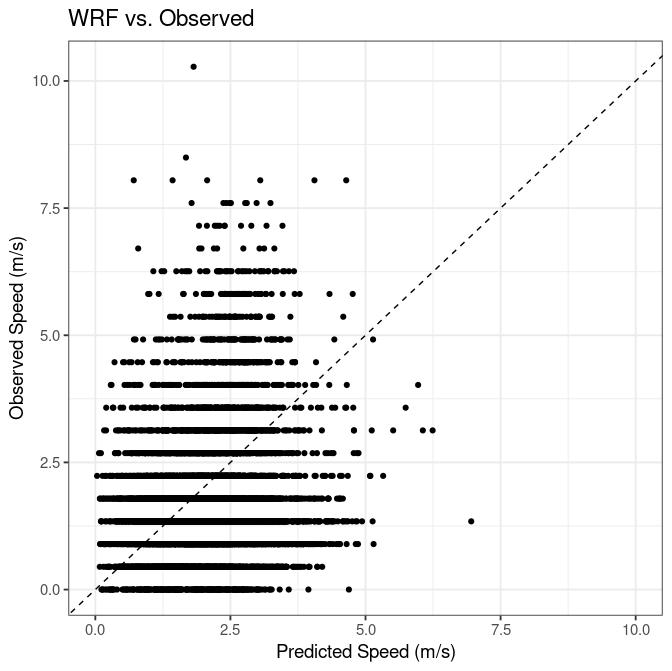
If this wind information will be used in a fire spread model (such as BEHAVE), we should ask if 10 minutes is the best averaging period to represent a quasi-steady state wind to drive that spread. Does the appropriate averaging period differ for simpler, single-layer fuels like grass, versus multi-layer, heavier fuels like a forest with an understory and a separate canopy? How long does a lofted ember get carried by the wind, and is it closer to the gust period or the averaging period? Overall, can one mean wind speed and direction, and one gust speed answer all these questions to the needed level of accuracy? Are there better ways to represent wind, besides a mean and a measure of gust speed? How large an area can reliably be represented by one observation location, and what factors determine that?
WIND-BASED FIRE QUESTIONS AND CALCULATIONS
Logically, many fire behavior tools rely on wind speed and direction as input variables. All the Rothermel-based tools, such as BEHAVE, FARSITE, and FSPro rely on wind speed to compute rate of spread and sometimes spotting distance. The wind used for rate of spread can be eye-level, 20 feet, or 10 meters above-ground, any of which are supposed to be adjusted to midflame height. The physical spotting process and any spotting computations depend on wind speed at and above the height from which the ember was released, often considered canopy height, where winds would affect ember transport. Some of these tools only use mean wind for their computations, but practitioners sometimes apply estimated gust speeds, to assess what could happen under those conditions.
Using these tools often requires the analyst to apply a more or less ad hoc wind adjustment factor (WAF). WAFs convert a measured or forecast wind at some height to an estimated midflame wind speed for fire spread models; they represent the effects of differences in height between midflame height and an observation or model forecast height, the influence of vegetative structure, and the role of terrain sheltering. Most common WAFs are between 0.2 and 0.6, with single-digit precision. Does wind need to be any more precise? What are the consequences if a user selects 0.4 but a value of 0.5 would have been equally or more valid?
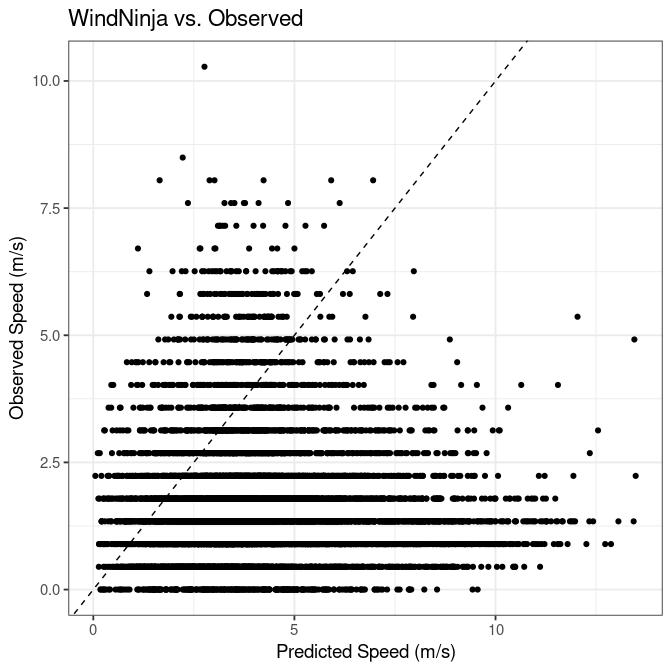
We have framed these questions in the context of existing tools and mental models of wind-fire interactions. There are many new tools being developed, or that could be developed, if we build newer mental models. Those models need to be consistent with the time and space scales of fire management and fire physics, as noted earlier. And sampling intervals for observations to feed these tools must be consistent with those scales, processes, and needs. The tools can also exploit the significant advances in technology that have occurred since the existing tools and models were first developed.
MODELING WIND
Most weather forecast models operate on a grid, with a fixed spacing between the center of the grid boxes. This spacing can be anywhere from about 0.5 miles to 30 miles (1 km to 50 km), depending on the model. The wind speed and direction at any location and forecast time are, in simplistic terms, the values necessary to conserve mass and momentum in that cell and among its neighbors. They are essentially averages over the entire volume of that cell at a given moment in time.
As such, they are not always representative of the wind at a given point within that cell (Figure 1) at a given instant. Because of cell size, model wind speed and direction change much more slowly over a few seconds or minutes than do observed wind speed and direction at any point in the cell. Downscaling –finding a reliable or robust conversion relationship between a cell and a point – is not straightforward.
Comparing a collection of synchronous values from the cell and the point can sometimes provide a statistical relationship, but as illustrated in Figure 1, that isn’t always successful. Some downscaling methods, like WindNinja, seek to use faster, simplified versions of the same physical laws used in more complex weather forecast models but they are not always successful at this either (Figure 2).
Operational analysts and research scientists spend a great deal of effort improving fire behavior models. Yet those improvements are less effective if the wind information they rely on is unrepresentative or poorly defined. Fire and smoke applications are sensitive to wind variations on spatial and temporal scales that are not always represented well by conventions developed to support maritime or aviation needs or dictated by instrument design and limitations.
Some fire behavior analysts and operational meteorologists are already aware of one or more of the issues outlined above; they have work-around solutions that only they know, which improve upon the standard systems. Other fire practitioners may have no idea how weakly wind forecasts or observations relate to what they will actually see on a prescribed fire or wildfire.
While we can never eliminate the need to interpret modeled winds as they relate to the real world, and we will certainly never replace direct personal experience, there are definitely areas in which research can decrease the amount of guesswork needed to render wind forecasts useful for fire management. We can also improve how we teach students of fire about wind forecasts and observations. There is a clear opportunity to reframe wind more suitably for wildland fire concerns and to use modern technology and computational resources to optimize what models and tools we make.
Revisiting definitions of wind and gusts with fire and smoke in mind will help clear the path toward future advances and successes. Hopefully, the thoughts presented here will spark conversations among practitioners and scientists about wind, and perhaps some new ideas or tools to fill in these knowledge gaps.
WORKSHOP PARTICIPANTS
The participants at the Minneapolis workshop included the authors and the following: Andrew M. Chiodi, Cooperative Institute for Climate and Ecosystem Studies, University of Washington, and National Oceanographic and Atmospheric Administration, Pacific Marine Environmental Laboratory, Seattle, WA.; IAWF board member Scott Goodrick, USDA Forest Service, Southern Research Station, Athens, Georgia; Mike Griesinger, National Weather Service Forecast Office, Chanhassen, Minnesota; Nick Nauslar, fire science and operations officer at the NOAA/NWS Storm Prediction Center, National Weather Service, Boise, Idaho; Christoper Rodell, University of British Columbia, Vancouver; Julia Ruthford, USDA Forest Service Fire and Aviation Management Northern California Coordination Center, Redding; Alan Srock, St Cloud State University, St. Cloud, Minnesota; Natalie Wagenbrenner, USDA Forest Service, Rocky Mountain Research Station.
Brian Potter is a research meteorologist with the US Forest Service, studying fire-atmosphere interactions for the US Forest Service since 1994. He serves on the National Wildfire Coordinating Group’s Fire Weather Subcommittee, the Interagency Wildfire Air Quality Program’s Air Resource Advisor Teaching Cadre, and the Pacific Northwest Region’s Teaching Cadre for Advanced Fire Behavior Calculations. Potter was a member of the USDA Forest Service National Prescribed Fire Program Review team. His past research has focused on atmospheric processes associated with wildfire growth, and his current research continues that, but focuses increasingly on weather needs for safe and successful prescribed burning.
Kara Yedinak has been a materials research engineer with the USDA Forest Service since 2018. Yedinak has a background in physics and engineering science, and an interest in investigating the fundamentals of fire science across a wide range of applications. She works in the field, as well as in the laboratory. Yedinak’s research interests include fire acoustics, fire-vegetation-atmosphere interactions from millimeters up to hundreds of kilometers, plant and soil response to wildland fire, the application of chaos theory to fire propagation, and communityscale fire science in the wildland urban interface.
Joseph Charney has been a research meteorologist with the USDA Forest Service since 2001. As a research scientist, Charney uses numerical weather prediction models to study how weather conditions affect fire activity and smoke dispersion and investigates fire-atmosphere interactions at scales ranging from a few meters up to thousands of kilometers. Charney has served on the Fire Weather Subcommittee of the National Wildfire Coordinating Group (NWCG), and currently serves as executive secretary for the Interagency Council for Advancing Meteorological Services (ICAMS) Working Group on Fire Weather.